Complete Guide to Framing a Data Monetization Strategy
The size of data warehouses and data lakes of all the organizations are increasing with time. This could be due to increasing digital penetration or more capable technology. Context being there are lot of organizations that have huge amount of data but don’t know how to make the most out of it for the benefit of the organization. As a result of research through some papers and books, In this article we have mentioned processes which you should follow while framing your data monetization strategy. So lets directly dive into it
1. Discovery Phase
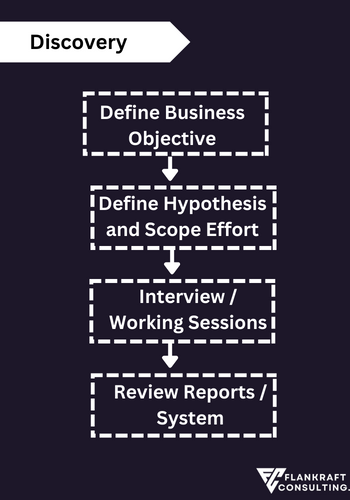
Here a hypothesis is formed which revolves around the business objective. Also the scope of the project and effort is predefined here. Once done with this, to get a better understanding of concepts, we need to arrange interviews and working session with the related departments or individuals. In the last stage of review reports we try to understand the current level of analytics implementation through available documentation and reports.
2. Decision Analysis Phase
The way a research scientist tries to understand the problem in the similar manner we need to understand the problem or opportunity through a continuous iterative cycle. This cycle could be broken down into four steps like Inform, diagnose, Action and Measure. At a base of this obvious comes data rather I would say quality data. The success of your data monetization efforts depend most on your ability to capture quality data. Let’s see the important steps in decision analysis phase
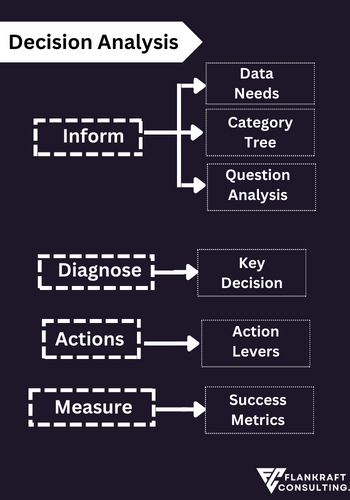
2.1 Inform
Here we try to convert the business problem statement in some kind of hypothesis. Doing this can help us align our further research to specific objectives. Once the hypothesis is framed it should be followed up with asking lot of question to drill down on root cause.
2.2 Diagnose
In this stage we finalize on the root cause and try to figure out future course of action. Where in Inform stage we are more focused on filtering of possible options in Diagnose stage we are focused on specificity to the selected option. Also different action levers are decided over here based on which execution will take place.
2.3 Action
This is the stage where main execution happens. We need to figure out our dependencies in execution. For example, if we want to implement a loyalty program we might need assistance of marketing department.
2.4 Measure
This could be one of the toughest stage. Measures could be of two types generally: direct and inferred responses. Direct means there is a proper arrangement of tracking the response of action. For example – Implementing web analytics solution to track button clicks on website. Inferred responses means there is no direct tagging between response and action, but it is inferred relationship. For example – if sales has gone down from last 6 weeks it could be probably be due to the price increase that we did l last month.
3. Monetization Strategy
The efforts in this phase are concurrent to decision analytics and agile analytics phase and employ data science and decision theory to structure the actions that guide you to the best decisions. Requirements from decision analytics phase are used to frame the strategy going forward.
The four important phases of Monetization Strategy are:
Business Levers, Decision analysis, Competitive Market & info, Guiding Principle.
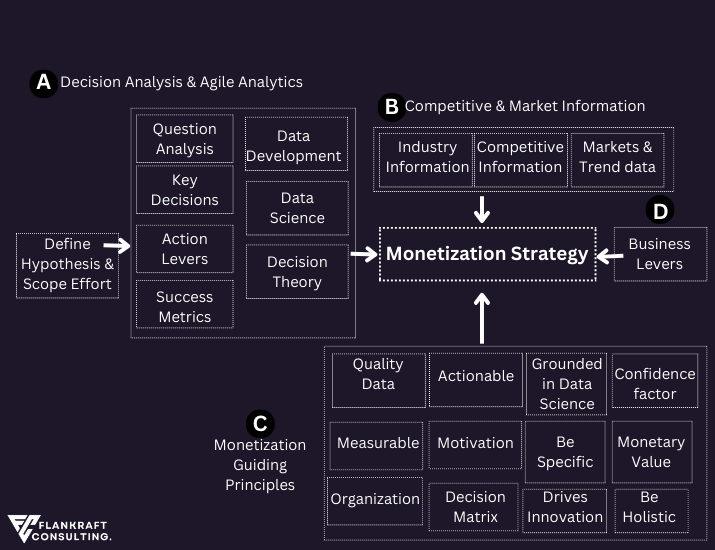
3.1 Business Levers
The main aim of any organization to build a monetization strategy could be to enable managers to take decisions that would increase revenue and reduced cost. Business levers is something that you will help you with the same. These levers can be something very specific to your business. The best way to figure out this is drill down more on your components in balance sheet.
3.2 Decision analysis & Agile Analytics
There are 8 processes that are involved in this phase. Some of the topics are self-explanatory and the rest are discussed in this article in this or that manner.
3.3 Competitive Market & Info
When it comes to competitive and market information there might be some variables which could be captured by company itself and some that cannot be. A holistic information should be gathered based on proprietary and third party data. Some of the important data points that could be considered are as follows
3.4 Guiding Principle
As we go ahead and make change sin the strategy there are certain elements which could act as metrics to continuously keep us on the track. We can see the 12 very important guiding principles in the above figure
4. Agile Analytics
4.1 Data development / Analytical Structure
This step involves preparation of good quality dataset that then can be used in analytics. It involves all the calculations, transformations and aggregations required to build a complete wholistic data set. It is not necessary that all sort of data will be available internally in the organization or will be available at single source. Ensuring that data correctly represents and is useful to the subject of analysis needs lot of effort which would be all considered in Data development step.
4.2 Data Science / Decision theory
With the help of descriptive and prescriptive analytics technique, data science helps you to generate and structure insights. The output of decision analysis phase and data development phase generally go into building the input of data science/decision science step. Data Science helps you to structure information into insights that are actionable. But we need something that can guide human to take decision as well, Decision theory comes in here as a solution here along with the blend of behavioural economics. This simply helps us to understand why we make certain choices and what are the trade-offs considered.
4.3 Guided Analytics
Guided Analytics is a combination of techniques in dashboard developments and User Interface (UI) / User Experience (UX). When it comes to experience delivered it can be divided into two types: Unguided and Guided. Unguided Experience is basically when analyst has a normal view of dashboard that allows him to identify events/news. Whereas in Guided experience you are provided with insights in opportunities and issues. Example – Sales dashboard of a tech company has revenue, profit, and cogs etc. generated region wise. This dashboard will provide you the information of the highest revenue/ profit generating regions. But would you be able to make an understanding on increase or decrease of sales compared to last month or year. Imagine that the dashboard has automatically data updated for last period for your comparison. This will simply allow you to have a guided view of analysis.
5. Enablement
This can be considered as the last phase in your data monetization strategy. Enablement phases is the iterative process and has to go through continuous improvement throughout the time. There are three things you need to figure out in this phase, namely: Testing, Training, Rollout.
One of the major part of testing is User Acceptance Testing (UAT). Here we make sure that the solution exactly solves the problem of the user that it was built for. Also, it is easy for user to adapt to the solution. To do so many a times we need to train our users on the solution. We need to tailor the programs to on-board users by training them this could be through different forms or combination of different forms namely: self-study, in-person, online classes etc. Following to this a controlled rollout of the analytics solution should be confirmed. Keeping users engaged should be one of the top priority while rolling out.
Understanding the above 5 phases of Monetization strategy of data you can surely push your revenue by leveraging opportunities through advanced analytics.